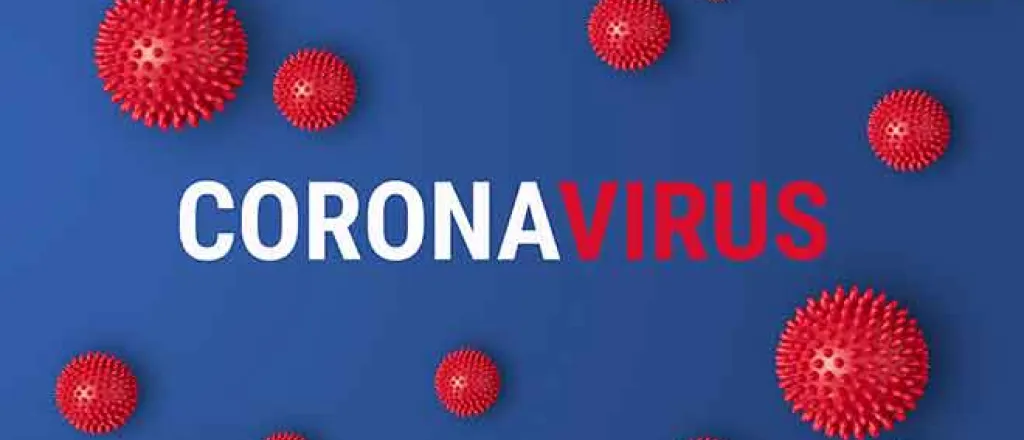
How much coronavirus testing is enough? States could learn from retailers as they ramp up
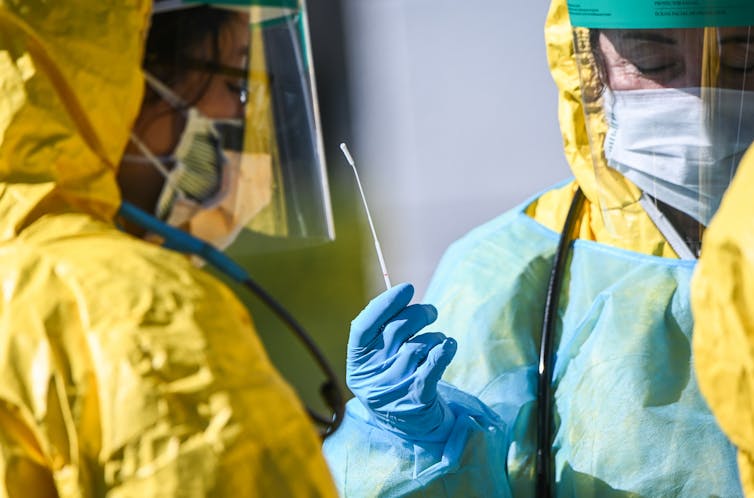
Siqian Shen, University of Michigan
As states develop plans to restart their economies, the big fear is that coronavirus cases will surge again. To keep the pandemic under control, strategic testing systems will be needed, and they will need to be scaled up fast.
But how many people should be tested? Who should be tested? And what should that testing system look like?
There isn’t one simple answer. The notion of “widespread testing” has a different meaning for big metropolitan areas, such as New York City and Detroit, than for rural areas like Montana or Alaska. For testing systems to be efficient, they need to be tailored to the demographics, circumstances and disease spread patterns for each.
As policymakers figure out the best design for each state or county, they could learn a lot from the retail industry, where strategic decisions such as where to locate warehouses and distribution centers are being made by companies like Amazon in the face of uncertain customer demand.
I have been researching complex systems design in health care, transportation and energy supply management and have found that good models using mathematics and data can help design such systems, even with the kind of information uncertainty we see with the new coronavirus spread.
It isn’t as simple as ‘test everyone’
Policymakers and researchers have floated several plans for reopening the country, with a range of ideas for how to conduct coronavirus testing. On one end of the spectrum, a plan sketched out by Nobel Prize-winning economist Paul Romer calls for 7% of the U.S. population to be tested at random daily. That’s roughly 23 million people every day.
Another plan, released Monday by a team coordinated by Harvard University’s center for ethics, recommended 5 million tests per day by early June and 20 million a day by August.
While random testing of that magnitude can provide a broad snapshot of the disease, it is not realistic for a country the size of the U.S. in the short term. Countries that are conducting random testing on large percentages of their populations, such as Singapore, Iceland and South Korea, have far fewer people and less complex demographics than the U.S.
The U.S. also has had serious challenges with its testing system, including a flawed diagnostic test approved by the CDC, scarce supplies for manufacturing test kits and running tests, and limited access to screening.
As of mid-April, U.S. public and corporate labs together were only testing about 150,000 coronavirus samples a day. In all, some 4 million tests had been conducted in the U.S., about 20% of them positive.
That high rate of positive tests, 20%, suggests that many COVID-19 cases probably aren’t being identified. The World Health Organization recommends conducting enough tests that no more than 10% come back positive. In the U.S., that would require more than 500,000 per day, according to recent estimates from Harvard public health researchers.
At this point, the U.S. needs to get the most value out of the limited testing capability it has. Some options include prioritizing states with the most infections and deaths from COVID-19; areas where health systems are overwhelmed; or groups that have the most contact with others, such as health workers and grocery store clerks.
Who should be tested?
One purpose of the mathematical models I work with is to help policymakers determine which population groups should be prioritized and how.
There are different reasons to prioritize different groups. For example, older adults and people with chronic illnesses have a higher likelihood of developing severe conditions if they get COVID-19. First responders, health workers, teachers and others who have close contact with large numbers of people also have a high chance of getting and spreading the disease. People living in close quarters such as nursing homes or prisons also run a high risk of infection.
Once priority groups are identified, models can show where to locate testing facilities and how to allocate the tests kits and other resources most efficiently.
Learning from retail
There is a large body of research that has traditionally been used to site retail stores, optimize inventory and production and manage stock levels as demand for products fluctuates by season.
Establishing a testing system for COVID-19 presents a similar set of challenges. The same mathematical models used to help retail companies like Amazon meet demand quickly for a wide range of products could incorporate test-manufacturing capacities, the needs of different populations and goals such as increasing testing speed and coverage.
One question is where to conduct COVID-19 testing. Another set of questions involves the capacity of those facilities, such as how many test kits they need and what their staffing levels should be to process enough tests while minimizing people’s travel distance.
There is one big point of uncertainty, and it’s the same question that widespread testing is helping to clarify: How will the disease spread?
Retail companies know the demand patterns for most of their products quite well, given repeated orders and seasonal trends. But U.S. public health officials have only a few months of partial data on the spread and impact of this new coronavirus.
Adding to the complications, test results can create a biased picture of the pandemic depending on how many tests are done in each area and who was tested.
An iterative process is necessary to learn to analyze the data and predict the disease’s spread.
Whether the U.S. can scale up testing fast enough to keep the coronavirus spread under control mainly relies on strategic testing capabilities and how well we can design that testing system.
[Get facts about coronavirus and the latest research. Sign up for The Conversation’s newsletter.]
Siqian Shen, Associate Professor of Industrial and Operations Engineering, University of Michigan
This article is republished from The Conversation under a Creative Commons license. Read the original article.